PyODPS は MaxCompute の Python SDK です。 MaxCompute オブジェクトに対する基本操作と DataFrame フレームワークをサポートし、MaxCompute でのデータ分析を容易にします。すべてのインターフェイスとクラスの詳細は、「GitHub プロジェクト」とPyODPS ドキュメントをご参照ください。
-
開発者は PyODPS のエコロジカルな開発に参加するよう招待されます。 詳細は、GitHub ドキュメントをご参照ください。
-
PyODPS エコ成長を加速するため、開発者は問題を送信してリクエストをマージすることもできます。 詳細は、コードをご参照ください。
-
DingTalk テクノロジー交流グループ : 11701793
PyODPS のインストール
PyODPS は Python 2.6 以降のバージョンをサポートしています。 システムに PIP をインストール後、 pip install pyodps
を実行する必要があります。 PyODPS の関連する依存関係は自動でインストールされます。
クイックスタート
from odps import ODPS
odps = ODPS('**your-access-id**', '**your-secret-access-key**', '**your-default-project**',
endpoint='**your-end-point**')
初期化が完了したら、テーブル、リソース、および関数を操作できます。
プロジェクト
プロジェクトは、データベース同様、MaxCompute の基本的な操作単位です。
get_project
を呼び出してプロジェクトを取得します (以下のコードを参照)。
project = odps.get_project('my_project') # Obtain a project.
project = odps.get_project() # Obtain the default project.
- パラメータが入力されていない場合は、デフォルトのプロジェクトを使用します。
-
exist_project
を呼び出して、プロジェクトが存在するかどうかチェックできます。 -
テーブルは MaxCompute のデータ格納単位です。
テーブル操作
list_tables
を呼び出して、プロジェクト内のすべてのテーブルを一覧表示します (以下のコードを参照)。
for table in odps.list_tables():
# Process each table
exist_table
を呼び出して、テーブルが存在するかどうかチェックし、get_table を呼び出してテーブルを取得します。
t = odps.get_table('dual')
t.schema
odps.Schema {
c_int_a bigint
c_int_b bigint
c_double_a double
c_double_b double
c_string_a string
c_string_b string
c_bool_a boolean
c_bool_b boolean
c_datetime_a datetime
c_datetime_b datetime
}
t.lifecycle
-1
print(t.creation_time)
2014-05-15 14:58:43
t.is_virtual_view
False
t.size
1408
t.schema.columns
[<column c_int_a, type bigint>,
<column c_int_b, type bigint>,
<column c_double_a, type double>,
<column c_double_b, type double>,
<column c_string_a, type string>,
<column c_string_b, type string>,
<column c_bool_a, type boolean>,
<column c_bool_b, type boolean>,
<column c_datetime_a, type datetime>,
<column c_datetime_b, type datetime>]
テーブルのスキーマの作成
- テーブル列とオプションのパーティションを介して初期化します (以下のコードを参照)。
from odps.models import Schema, Column, Partition columns = [Column(name='num', type='bigint', comment='the column')] partitions = [Partition(name='pt', type='string', comment='the partition')] schema = Schema(columns=columns, partitions=partitions) schema.columns [<column num, type bigint>, <partition pt, type string>]
- 初期化は
Schema.from_lists
を呼び出すほうが簡単ですが、列とパーティションのアノテーションを直接設定することができません。schema = Schema.from_lists(['num'], ['bigint'], ['pt'], ['string']) schema.columns [<column num, type bigint>, <partition pt, type string>]
テーブルの作成
table = odps.create_table('my_new_table', schema)
table = odps.create_table('my_new_table', schema, if_not_exists=True) # Create a table only when no table exists.
table = o.create_table('my_new_table', schema, lifecycle=7) # Set the life cycle.
>>> # Create a non-partition table.
>>> table = o.create_table('my_new_table', 'num bigint, num2 double', if_not_exists=True)
>>> # To create a partition table, you can input (list of table fields, list of partition fields).
>>> table = o.create_table('my_new_table', ('num bigint, num2 double', 'pt string'), if_not_exists=True)
関連設定がない場合、テーブル作成時に使用できるデータ型は BIGINT、DOUBLE、DECIMAL、STRING、DATETIME、BOOLEAN、MAP、および ARRAY だけです。
options.sql.use_odps2_extension = True
を設定して新しい型を有効にすることができます (以下のコードを参照)。
from odps import options
options.sql.use_odps2_extension = True
table = o.create_table('my_new_table', 'cat smallint, content struct<title:varchar(100), body string>')
テーブルデータの取得
- 以下の方法で
head
を呼び出して、テーブルデータ (取得できるデータレコードは各テーブルの最初の 10,000 個以下) を取得します。>>> t = odps.get_table('dual') >>> for record in t.head(3): >>> print(record[0]) # Obtain the value at the zero position. >>> print(record['c_double_a']) # Obtain a value through a field. >>> print(record[0: 3]) # Slice action >>> print(record[0]) # Obtain values at multiple positions. >>> print(record['c_int_a', 'c_double_a']) # Obtain values through multiple fields.
- テーブル上で
open_reader
を実行してリーダーを開き、データを読み取ります。WITH 式を使用できます。# Use the with expression. with t.open_reader(partition='pt=test') as reader: count = reader.count for record in reader[5:10] # This action can be performed multiple times until a certain number (indicated by count) of records are read. This statement can be transformed to parallel action. # Process a record. # Do not use the with expression. reader = t.open_reader(partition='pt=test') count = reader.count for record in reader[5:10] # Process a record.
- Tunnel API を呼び出してテーブルデータを読み取ります。
open_reader
操作は、Tunnel API にカプセル化されています。
データのを書き込み
テーブルオブジェクトは、open_writer
操作を実行して、ライターを開き、データを書き込むことができます (open_reader
とほぼ同じ)。
# Use the with expression.
with t.open_writer(partition='pt=test') as writer:
writer.write(records) # Here, records can be any iteratable records and are written to block 0 by default.
with t.open_writer(partition='pt=test', blocks=[0, 1]) as writer: # Open two blocks at the same time
writer.write(0, gen_records(block=0))
writer.write(1, gen_records(block=1)) # The two write operations can be parallel in multiple threads. Each block is independent.
# Do not use the WITH expression.
writer = t.open_writer(partition='pt=test', blocks=[0, 1])
writer.write(0, gen_records(block=0))
writer.write(1, gen_records(block=1))
writer.close() # You must close the writer. Otherwise, the written data may be incomplete.
同様に、テーブルへのデータの書き込みは Tunnel API にカプセル化されています。 詳細は、「データのアップロードとダウンロードチャネル」をご参照ください。
テーブルの削除
odps.delete_table('my_table_name', if_exists=True) # Delete a table only when the table exists
t.drop() # The drop function can be directly executed if a table object exists.
テーブルのパーティション化
- 基本操作
テーブルのすべてのパーティションを走査するコードを以下に示します。
for partition in table.partitions: print(partition.name) for partition in table.iterate_partitions(spec='pt=test'): Traverse list partitions.
パーティションが存在するかどうかチェックするコードを以下に示します。table.exist_partition('pt=test,sub=2015')
パーティションを取得するコードを以下に示します。partition = table.get_partition('pt=test') print(partition.creation_time) 2015-11-18 22:22:27 partition.size 0
- パーティションの作成
t.create_partition('pt=test', if_not_exists=True) # Create a partition only when no partition exists.
- パーティションの削除
t.delete_partition('pt=test', if_exists=True) # Delete a partition only when the partition exists. partition.drop() # Directly drop a partition if a partition object exists.
SQL
- SQL 文の実行
odps.execute_sql('select * from dual') # Run SQL in synchronous mode. ブロック化は SQL の実行が完了するまで続きます。 instance = odps.run_sql('select * from dual') # Run the SQL statements in asynchronous mode. instance.wait_for_success() # Blocking continues until SQL execution is completed.
- SQL 文の実行結果の読み取り
SQL 文を実行するインスタンスは、直接
open_reader
操作を実行できます。 1 番目のシナリオでは、以下のように SQL 文が構造化データを返します。with odps.execute_sql('select * from dual').open_reader() as reader: for record in reader: # Process each record.
desc
など、SQL で実行する可能性がある操作により reader.raw
属性を介して未加工の SQL 実行結果を取得します (以下のコードを参照)。
with odps.execute_sql('desc dual').open_reader() as reader:
print(reader.raw)
リソース
リソースは通常、MaxCompute 上の UDF および MapReduce に適用されます。
list_resources
を使用してすべてのリソースを一覧表示し、exist_resource
を使用して、リソースが存在するかどうかチェックします。 delete_resource
を呼び出してリソースを削除するか、リソースオブジェクトの drop
メソッドを直接呼び出すことができます。
PyODPS は主にファイルリソースとテーブルリソースの 2 つのリソースタイプをサポートします。
- ファイルリソース
ファイルリソースには、基本的な
file
タイプ、およびpy
、jar
、およびarchive
が含まれます。説明 DataWorks では、py 形式のファイルリソースはファイルとしてアップロードする必要があります。 詳細は、「Python UDF」をご参照ください。ファイルリソースの作成リソース名、ファイルタイプ、およびファイルライクオブジェクト (または文字列オブジェクト) を指定して、ファイルリソースを作成します (以下のコードを参照)。resource = odps.create_resource('test_file_resource', 'file', file_obj=open('/to/path/file')) # Use a file-like object. resource = odps.create_resource('test_py_resource', 'py', file_obj='import this') # Use a string.
ファイルリソースの読み取りと変更ファイルリソースは、open
メソッドまたは MaxCompute エントリでopen_resource
を呼び出してファイルリソースを開くことができます。 開かれたオブジェクトはファイルライクオブジェクトです。 Pythonで構築されたopen
メソッドと同様、ファイルリソースもオープンモードをサポートしています。例 :with resource.open('r') as fp: # Open a resource in read mode. content = fp.read() # Read all content. fp.seek(0) # Return to the start of the resource. lines = fp.readlines() # Read multiple lines. fp.write('Hello World') # Error. Resources cannot be written in read mode. with odps.open_resource('test_file_resource', mode='r+') as fp: # Enable read/write mode. fp.read() fp.tell() # Current position fp.seek(10) fp.truncate() # Truncate the following content. fp.writelines(['Hello\n', 'World\n']) # Write multiple lines. fp.write('Hello World') fp.flush() # Manual call submits the update to MaxCompute.
以下のオープンモードをサポートしています。r
: 読み取りモードです。 ファイルを開くことはできますが書き込みはできません。w
: 書き込みモードです。 ファイルの書き込めはできますが、読み取りはできません。 ファイルを書き込みモードで開くと、最初にファイルの内容が消去されることにご注意ください。a
: 追加モードです。 内容をファイルの末尾に追加できます。r+
: 読み取り/書き込みモードです。 任意のコンテンツを読み書きできます。w+
:r+
とほぼ同じですが、最初にファイルの内容が消去されます。a+
:r+
とほぼ同じですが、書き込み中に限りファイルの末尾に内容を追加できます。
PyODPS では、ファイルリソースはバイナリモードで開くことができます。 たとえば、一部の圧縮ファイルはバイナリモードで開く必要があります。
rb
はバイナリ読み取りモードでファイルを開くこと、r+b
はバイナリ読み取り/書き込みモードでファイルを開くことを示します。 - テーブルリソース
テーブルリソースの作成
>>> odps.create_resource('test_table_resource', 'table', table_name='my_table', partition='pt=test')
テーブルリソースの更新>>> table_resource = odps.get_resource('test_table_resource') >>> table_resource.update(partition='pt=test2', project_name='my_project2')
DataFrame
PyODPS は、pandas と類似したインターフェイスを提供するDataFrame APIを提供しており、MaxCompute の計算機能を完全に利用することができます。 詳細は、「DataFrame」をご参照ください。
o = ODPS('**your-access-id**', '**your-secret-access-key**',
project='**your-project**', endpoint='**your-end-point**'))
ここでは、movielens 100K を例として使用します。 pyodps_ml_100k_movies
(動画関連のデータ)、pyodps_ml_100k_users
(ユーザー関連のデータ)、および pyodps_ml_100k_ratings
(格付け関連データ) という 3 つのテーブルが既に存在するとします。
from odps.df import DataFrame
users = DataFrame(o.get_table('pyodps_ml_100k_users'))
users.dtypes
head メソッドを使用して、データプレビュー用に最初の N 個のデータレコードを取得できます。
users.head(10)
user_id | age | sex | occupation | zip_code | |
0 | 1 | 24 | M | technician | 85711 |
1 | 2 | 53 | F | other | 94043 |
2 | 3 | 23 | M | writer | 32067 |
3 | 4 | 24 | M | technician | 43537 |
4 | 5 | 33 | F | other | 15213 |
5 | 6 | 42 | M | executive | 98101 |
6 | 7 | 57 | M | administrator | 91344 |
7 | 8 | 36 | M | administrator | 05201 |
8 | 9 | 29 | M | student | 01002 |
9 | 10 | 53 | M | lawyer | 90703 |
特定のフィールドだけを表示するには、フィールドにフィルターを追加します。
users[['user_id', 'age']].head(5)
user_id | age | |
0 | 1 | 24 |
1 | 2 | 53 |
2 | 3 | 23 |
3 | 4 | 24 |
4 | 5 | 33 |
複数のフィールドを除外することもできます。
users.exclude('zip_code', 'age').head(5)
user_id | Sex | Occupation | |
0 | 1 | M | Technician |
1 | 2 | F | Other |
2 | 3 | M | Writer |
3 | 4 | M | Technician |
4 | 5 | F | Other |
特定のフィールドを除外し、計算によって新しい列を取得する場合は、次の例に示すコードを使用します。
たとえば、性別が Male (男) の場合、sex_bool 属性を追加して True に設定します。 Male でない場合は、False に設定します。
users.select(users.exclude('zip_code', 'sex'), sex_bool=users.sex == 'M').head(5)
user_id | Age | Occupation | sex_bool | |
0 | 1 | 24 | Technician | True |
1 | 2 | 53 | Other | False |
2 | 3 | 23 | Writer | True |
3 | 4 | 24 | Technician | True |
4 | 5 | 33 | Other | False |
users.age.between(20, 25).count().rename('count')
943
users.groupby(users.sex).count()
Sex | Count | |
0 | Female | 273 |
1 | Male | 670 |
ユーザーを仕事で分割するには、人数が多い順に上位 10 の仕事を取得し、人数の降順で仕事をソートします。
例 :
>>> df = users.groupby('occupation').agg(count=users['occupation'].count())
>>> df.sort(df['count'], ascending=False)[:10]
Occupation | Count | |
0 | Student | 196 |
1 | Other | 105 |
2 | Educator | 95 |
3 | Administrator | 79 |
4 | Engineer | 67 |
5 | Programmer | 66 |
6 | Librarian | 51 |
7 | Writer | 45 |
8 | Executive | 32 |
9 | Scientist | 31 |
users.occupation.value_counts()[:10]
Occupation | Count | |
0 | Student | 196 |
1 | Other | 105 |
2 | Educator | 95 |
3 | Administrator | 79 |
4 | Engineer | 67 |
5 | Programmer | 66 |
6 | Librarian | 51 |
7 | Writer | 45 |
8 | Executive | 32 |
9 | Scientist | 31 |
%matplotlib inline
users['occupation'].value_counts().plot(kind='barh', x='occupation',
ylabel='prefession')
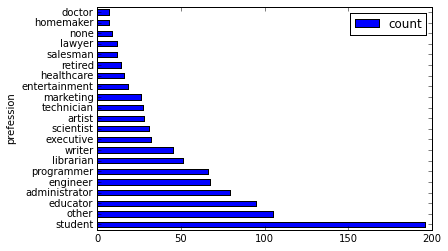
users.age.hist(bins=30, title="Distribution of users' ages", xlabel='age', ylabel='count of users')
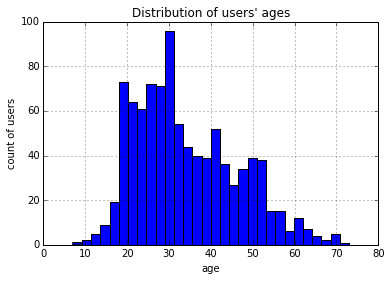
JOIN を使用して 3 つのテーブルを結合し、結合したテーブルを新しいテーブルとして保存します。
movies = DataFrame(o.get_table('pyodps_ml_100k_movies'))
ratings = DataFrame(o.get_table('pyodps_ml_100k_ratings'))
o.delete_table('pyodps_ml_100k_lens', if_exists=True)
lens = movies.join(ratings).join(users).persist('pyodps_ml_100k_lens')
lens.dtypes
odps.Schema {
movie_id int64
title string
release_date string
video_release_date string
imdb_url string
user_id int64
rating int64
unix_timestamp int64
age int64
sex string
occupation string
zip_code string
}
labels = ['0-9', '10-19', '20-29', '30-39', '40-49', '50-59', '60-69', '70-79']
cut_lens = lens[lens, lens.age.cut(range(0, 81, 10), right=False, labels=labels).rename('age group')]
>>> cut_lens['age group', 'age'].distinct()[:10]
Age-group | Age | |
0 | 0-9 | 7 |
1 | 10-19 | 10 |
2 | 10-19 | 11 |
3 | 10-19 | 13 |
4 | 10-19 | 14 |
5 | 10-19 | 15 |
6 | 10-19 | 16 |
7 | 10-19 | 17 |
8 | 10-19 | 18 |
9 | 10-19 | 19 |
cut_lens.groupby('age group').agg(cut_lens.rating.count().rename('total rating'), cut_lens.rating.mean().rename('average rating'))
Age-group | Average rating | Total rating | |
0 | 0-9 | 3.767442 | 43 |
1 | 10-19 | 3.486126 | 8181 |
2 | 20-29 | 3.467333 | 39535 |
3 | 30-39 | 3.554444 | 25696 |
4 | 40-49 | 3.591772 | 15021 |
5 | 50-59 | 3.635800 | 8704 |
6 | 60-69 | 3.648875 | 2623 |
7 | 70-79 | 3.649746 | 197 |
設定
odps.options
を介して取得可能な一連の設定オプションを提供します。以下は、設定可能な MaxCompute オプションの一覧です。
- 一般的な設定
オプション 説明 デフォルト値 end_point MaxCompute エンドポイント なし default_project デフォルトプロジェクト なし log_view_host LogView ホスト名 なし log_view_hours LogView 保持時間 (1 時間単位) 24 local_timezone 使用されているタイムゾーン。 True は現地時間、False は UTC を示します。 pytz のタイムゾーンも使用できます。 1 lifecycle すべてのテーブルのライフサイクル なし temp_lifecycle 一時テーブルのライフサイクル 1 biz_id ユーザー ID なし verbose ログを印刷するかどうか False verbose_log ログの受信者 なし chunk_size 書き込みバッファのサイズ 1496 retry_times 再試行のリクエスト回数 4 pool_connections 接続プール内のキャッシュ接続数 10 pool_maxsize 接続プールの最大容量 10 connect_timeout 接続タイムアウト 5 read_timeout 読み取りタイムアウト 120 completion_size オブジェクトの完全なリスト項目の数の上限 10 notebook_repr_widget 対話型グラフを使用します。 True sql.settings MaxCompute SQL でグローバルヒントを実行します。 なし sql.use_odps2_extension MaxCompute 2.0 の言語拡張を有効にします。 False - データのアップロード/ダウンロード設定
オプション 説明 デフォルト値 tunnel.endpoint Tunnel エンドポイント なし tunnel.use_instance_tunnel 実行結果を取得するには、Tunnel インスタンスを使用します。 True tunnel.limited_instance_tunnel Tunnel インスタンスによって取得される結果の数を制限します。 True tunnel.string_as_binary 文字列型では Unicode ではなくバイトを使用します。 False - DataFrame の設定
オプション 説明 デフォルト値 interactive インタラクティブ環境にあるかどうか。 検出値に応じます。 df.analyze MaxCompute 以外の組み込み関数を有効にするかどうか True df.optimize DataFrame 全体の最適化を有効にするかどうか True df.optimizes.pp DataFrame 述語のプッシュ最適化を有効にするかどうか True df.optimizes.cp DataFrame 列の調整最適化を有効にするかどうか True df.optimizes.tunnel DataFrameトンネル最適化を有効にするかどうか True df.quote MaxCompute SQL 末尾のフィールドとテーブル名のマーキングに `` を使うかどうか True df.libraries 実行中の DataFrame に使用されるサードパーティのライブラリ (リソース名) なし - PyODPS ML の設定
オプション 説明 デフォルト値 ml.xflow_project デフォルトの Xflow プロジェクト名 algo_public ml.use_model_transfer モデル PMML の取得に ModelTransfer を使用するかどうか。 True ml.model_volume ModelTransfer 使用時に使用されるボリューム名 pyodps_volume