About polymorphic tiered storage
Background
With the rapid development of digitization, the need to efficiently process large-scale, multimodal, and polymorphic data of all types emerges from various industries. Traditional middleware-based solutions are struggling to keep up with the high demands of spatial computing performance. To improve the efficiency of retrieving massive data of all types and bring spatial data processing online, GanosBase natively supports a variety of storage types for spatial data and numerous computing operators. It significantly improves the query performance by pushing down spatial computing. With the improvements of computing efficiency, the rapidly growing size of spatial data and objects can lead to high resource costs. To face the challenge of balancing costs and efficiency, enterprises have to take some businesses offline periodically or even completely. This leads to frequent switches of storage media during business development and increases O&M and R&D costs. Therefore, the GanosBase team spent over two years developing a spatical data management solution that is cost-effective and easy-to-use. The GanosBase team focuses on the following core areas for feature planning and development:
Use cost-effective storage media (not in the form of external table) to reduce costs.
Minimize query performance loss while using cost-effective storage media.
Manage and utilize data across multiple storage media with transparency.
The GanosBase team developed the polymorphic tiered storage of spatial data based on PolarDB for PostgreSQL (Compatible with Oracle) 2.0. Polymorphic tiered storage is a cost-effective spatial data management solution, which can also be used to manage general database field types, such as BLOB
, TEXT
, JSON
, JSONB
, and ANYARRAY
.
Overview
Polymorphic tiered storage provides better read and write performance at much lower storage costs than the traditional tiered hot/cold storage. It supports tiered storage for hot and cold data and is compatible with multiple object storage media such as OSS, MinIO, and HDFS. It offers flexible storage combinations for large object types and spatial data types. Currently, this feature only supports OSS. For more information, see Overview.
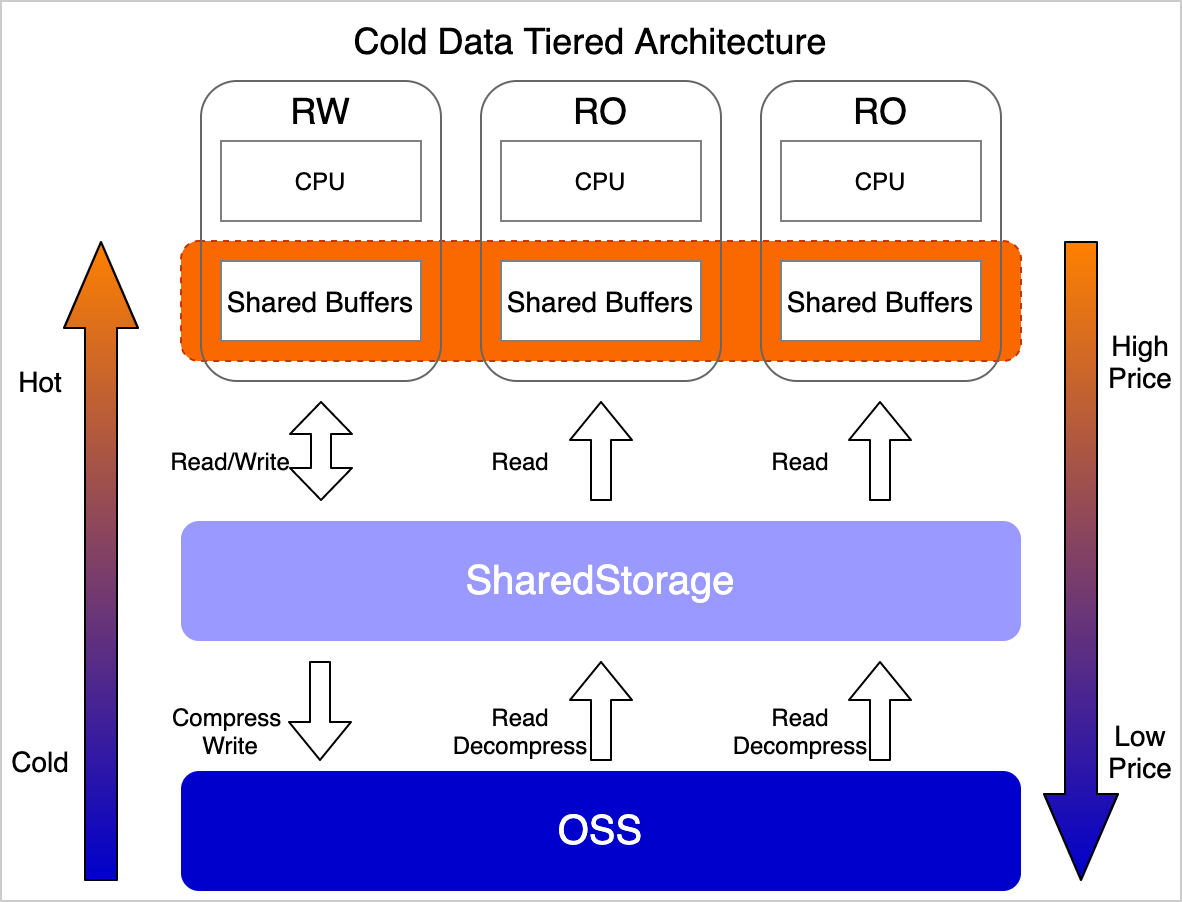
With the polymorphic tiered storage feature, you can execute simple SQL statements to transfer expired data, large object data, and spatial data to OSS, enabling elastic, cost-effective, and reliable data management. After the transfer, you can execute SQL statements to access the data without any rewriting. This allows you to perform complex operations such as CRUD operations and table joins with full transparency. Additionally, if the data access frequency increases, the materialized cache can be dynamically adjusted to provide the same access performance as database disks.
Technical advantages
Polymorphic tiered storage offers the following technical advantages:
Cost: Supports data compression with an average compression ratio of 50%, and in some cases, up to 20%, reducing 90% or more of the original costs.
Performance: The materialized cache layer accelerates cold data access with a 20%-80% performance loss.
Ease of use: Hot and cold data is stored in tiers. You can execute SQL statements to perform CRUD operations on data in cold storage without any rewriting.
Reliability: Leverages OSS to provide high reliability. You can create snapshots for cold data without consuming additional storage. The snapshot data can be used for quick restoration.
Flexibility: Supports tiered hot/cold storage to store tables, large objects, and partitions in OSS.
Cost
The volume of spatio-temporal data is now increasing explosively. As the data volume increases to terabytes or even petabytes, the access frequency of historical or auxiliary data decreases. As a result, reducing storage costs becomes a core customer requirement. In the
PolarDB for PostgreSQL (Compatible with Oracle) tiered hot/cold storage architecture, cold data is segmented into small blocks, compressed, and then stored in OSS. This achieves a general data compression ratio of 20%-40%, a spatio-temporal data compression ratio of 60%-70%, and an average compression ratio of 50%. This process effectively reduces the data volume by 50% when data is transferred from database disks to OSS, ultimately reducing storage costs by 90% or even more. The following table compares PolarDB disks with OSS in aspects such as billing rules, compression ratio, and the monthly storage fees for 100 GB of data:
| Disk | Polymorphic tiered storage (data stored in OSS) |
| Disk | Polymorphic tiered storage (data stored in OSS) |
Billing rules | PSL4USD 0.238 per GB-month | |
Compression ratio | 0 | 50% |
Storage fees for 100 GB of data | 0.238*100 GB=USD 23.8 | LRS: Total USD 1.103. ZRS: Total USD 1.398.
|
The storage fees of OSS are less than 10% of that of database disks.
Performance
Typically, the latency of accessing data in OSS is much higher than that on database disks. The performance of direct read and write operations on data stored in OSS is low. The
PolarDB for PostgreSQL (Compatible with Oracle) polymorphic tiered storage feature leverages database disks to provide a materialized cache layer for data stored in OSS. This achieves automatic tiered storage based on the access patterns of data blocks. Read and write operations can access data in the materialized cache first to ensure performance. The overall performance of update and insert operations is 90% of that on database disks. The point query performance is 80% of that on database disks. The lifecycle of data in the materialized cache is determined by access frequency. This ensures high access performance while reducing storage costs.
Ease of use
The PolarDB for PostgreSQL (Compatible with Oracle) polymorphic tiered storage feature offers a transparent tiered hot/cold storage solution that is easy to use. Complex operations such as CRUD operations, index scans, and join queries are supported for data stored in OSS with no modifications required for SQL statements. Additionally, you can transfer data with ease. For example, you can create rules to automatically transfer partitioned tables to OSS, or transfer base tables and indexes to OSS with a few clicks. For more information, see Best practices.
Reliability
OSS provides 99.9999999999% data reliability and 99.995% data availability. It also features LRS and ZRS to ensure data reliability. However, you still need to perform backup and restoration on data in OSS. Even with low update and access frequencies, the data in OSS is still required for critical query analysis and data mining tasks in business scenarios. In case of accidental deletion or modification, it is essential to be able to quickly restore the data. The PolarDB for PostgreSQL (Compatible with Oracle) polymorphic tiered storage feature supports backup and restoration of cold data. It implements data versioning based on the copy-on-write (COW) mechanism and can take a snapshot in seconds. After the first snapshot is created, replicas are created for updated data blocks only. This ensures data reliability at minimal cost.
Flexibility
The PolarDB for PostgreSQL (Compatible with Oracle) polymorphic tiered storage feature supports various hot and cold storage combinations:
Store data of the entire table in OSS and retain indexes on disks to reduce costs while maintaining access performance.
Store large and auxiliary fields in OSS and retain the remaining fields on disks.
Store expired partitions in OSS and retain hot partitions on disks, which is a typical data tiering mode. This approach can be further customized, such as storing cold partitions and indexes in OSS, storing hot partition data in OSS but retaining indexes on disks, and storing hot partitions entirely on disks to ensure query performance.
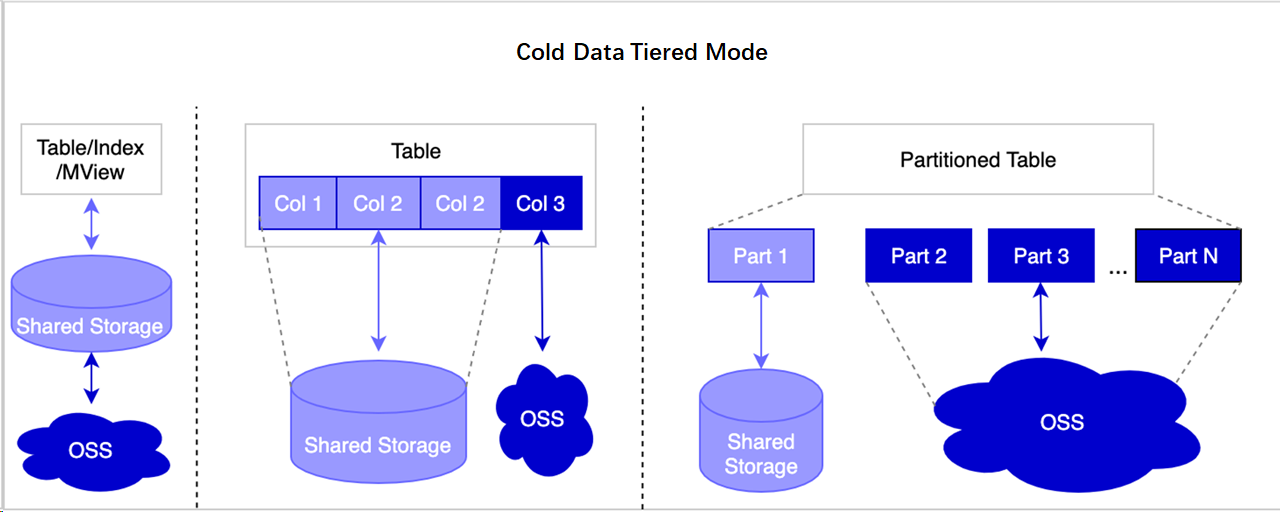
Best practices
Case 1: Automatically archive expired partitions to cold storage
Background
Trajectory data is stored in a table partitioned by month. Over time, the frequency of accessing the data generated three months ago significantly decreases. To reduce storage costs, it is necessary to automatically archive partitions created three months ago to cold storage.
Procedure
Create a test database. For more information, see Create a database. Execute the following statements to create a partitioned table and insert test data to the table:
CREATE TABLE traj(
tr_id serial,
tr_lon float,
tr_lat float,
tr_time timestamp(6)
)PARTITION BY RANGE (tr_time);
CREATE TABLE traj_202301 PARTITION OF traj
FOR VALUES FROM ('2023-01-01') TO ('2023-02-01');
CREATE TABLE traj_202302 PARTITION OF traj
FOR VALUES FROM ('2023-02-01') TO ('2023-03-01');
CREATE TABLE traj_202303 PARTITION OF traj
FOR VALUES FROM ('2023-03-01') TO ('2023-04-01');
CREATE TABLE traj_202304 PARTITION OF traj
FOR VALUES FROM ('2023-04-01') TO ('2023-05-01');
INSERT INTO traj(tr_lon,tr_lat,tr_time) values(112.35, 37.12, '2023-01-01');
INSERT INTO traj(tr_lon,tr_lat,tr_time) values(112.35, 37.12, '2023-02-01');
INSERT INTO traj(tr_lon,tr_lat,tr_time) values(112.35, 37.12, '2023-03-01');
INSERT INTO traj(tr_lon,tr_lat,tr_time) values(112.35, 37.12, '2023-04-01');
CREATE INDEX traj_idx on traj(tr_id);
Create the polar_osfs_toolkit
extension in the test database to enable utility class functions. You can use the functions to archive primary tables and indexes, or partitioned tables to OSS with a few clicks.
CREATE extension polar_osfs_toolkit;
Connect to the postgres
database by using a privileged account to create the pg_cron extension. For more information, see pg_cron.
CREATE EXTENSION pg_cron;
Set up the scheduled task. Connect to the postgres
database by using a privileged account and create a task named task1 for the test database. This task invokes a stored procedure to automatically move partitions created three months ago to OSS. The task ID is returned.
SELECT cron.schedule_in_database('task1', '* * * * *', 'select polar_alter_subpartition_to_oss(''traj'', 3);', 'db01');
Sample result:
Check the execution results and historical execution records.
In this example, expired partitions are automatically moved to cold storage based on custom rules. The partitions in cold storage no longer occupy disk space, significantly reducing storage costs. CRUD operations can be performed with full transparency.
Case 2: Tiered storage of LOBs in a non-partitioned table
Background
Large objects (LOBs) are a set of data types such as BLOB
, TEXT
, JSON
, JSONB
, ANYARRAY
, and spatio-temporal data types. In this example, the LOBs of a table are separately stored in OSS and can be accessed with full transparency. This reduces costs through hot and cold data separation at the field level.
Procedure
Create a table containing LOBs. In this example, the TEXT
type is used. You can use the same method to process other data types.
CREATE TABLE blob_table(id serial, val text);
Specify the storage location for LOBs.
ALTER TABLE blob_table ALTER COLUMN val SET (storage_type='oss');
Insert data and verify storage location.
Insert data. At this point, all values of the val column are stored in OSS.
INSERT INTO blob_table(val) VALUES((SELECT string_agg(random()::text, ':') FROM generate_series(1, 100000)));
Check the storage location of the val column.
WITH tmp AS (SELECT 'pg_toast_'||b.oid||'_'||c.attnum AS tblname FROM pg_class b, pg_attribute c WHERE b.relname='blob_table' AND c.attrelid=b.oid AND c.attname='val') SELECT t.spcname AS storage_engine FROM pg_tablespace t, pg_class r, tmp m WHERE r.relname = m.tblname AND t.oid=r.reltablespace;
Sample result:
storage_engine
oss
(1 row)
Note
Only data inserted after you execute the ALTER COLUMN
statement is stored in OSS.
To transfer a LOB from an existing table to OSS, execute the ALTER COLUMN
SQL statement first to set the storage location for LOBs. Then execute the VACUUM FULL
statement to rewrite the data. If you do not execute the VACUUM FULL
statement, historical data remains on disks and only new data is stored in OSS. Executing this statement does not affect the CRUD operations on the table. However, if the data volume is large, the execution of the statement can be time-consuming, and the table is unavailable for read or write operations during the execution.
Case 3: Improve cost-effectiveness in spatio-temporal analysis scenarios
Spatio-temporal data analysis uses database capabilities for data mining and statistical analysis in scenarios involving spatial, spatio-temporal, and time series data. In this example, the statistical analysis of remote sensing images is used to demonstrate how polymorphic tiered storage can reduce storage costs while maintaining analysis performance.
Note
This example involves the usage of advanced features. You can skip intermediate steps and mainly focus on the final comparison results.
Background
The application of remote sensing images (raster data) in spatial business is growing. With the large volume of remote sensing data and its usage in multiple business areas, such as image browsing and statistical analysis, reducing storage costs and improving usability is particularly appealing for users who need to perform non-real-time statistical analysis. This example demonstrates how to use OSS to cost-effectively store remote sensing images and provide efficient statistical analysis features.
Procedure
Prepare data. In this example, four Landset remote sensing images are prepared.
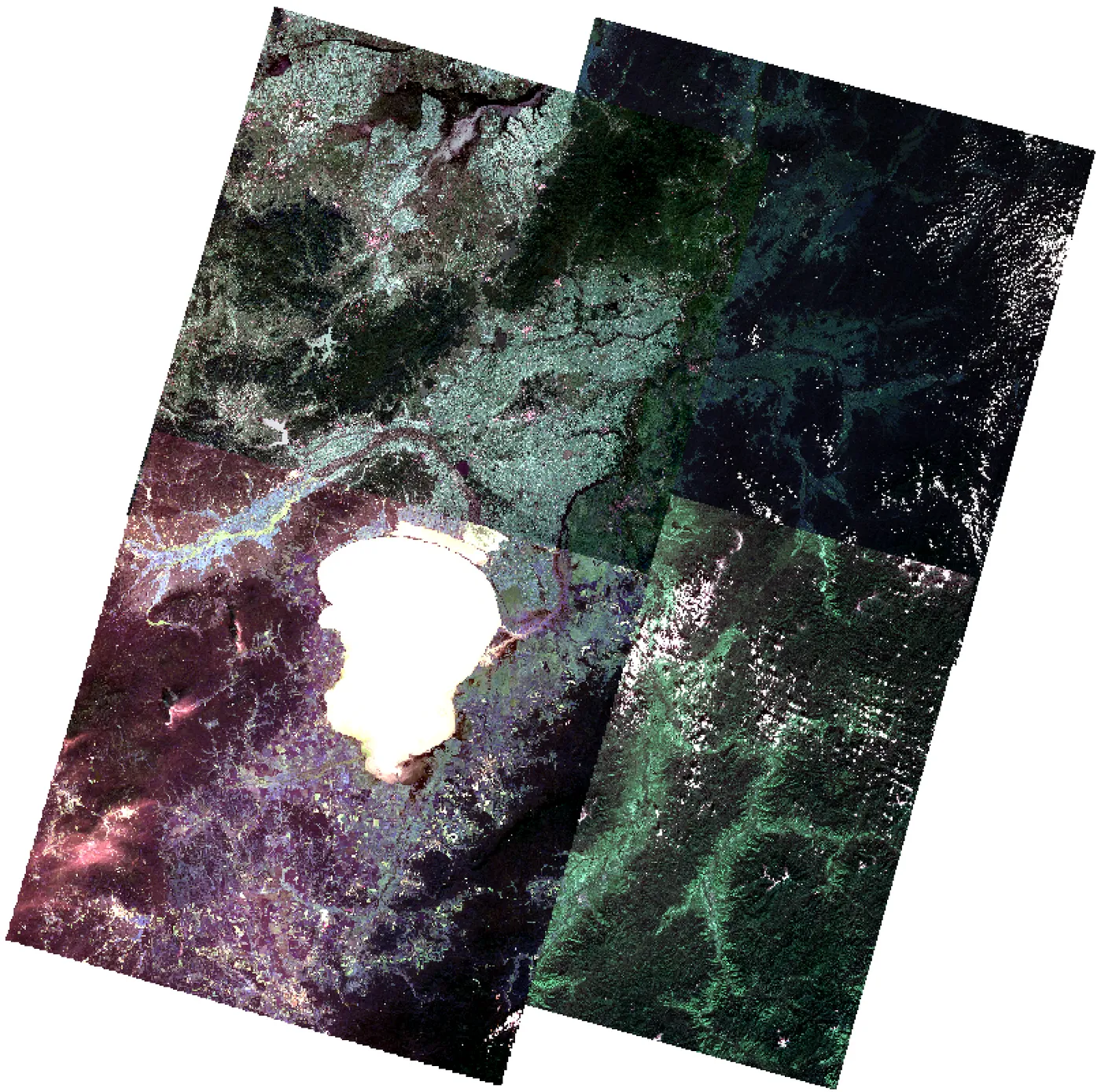
Import remote sensing images into the database.
Create a test database named rastdb.
Create the ganos_raster extension in the test database. For more information, see GanosBase Raster.
CREATE EXTENSION ganos_raster CASCADE;
Import images.
CREATE TABLE raster_table (id integer, rast raster);
INSERT INTO raster_table VALUES (1, ST_ImportFrom('rbt','/home/postgres/LC08_L1TP_113028_20190912_20190917_01_T1.TIF'));
INSERT INTO raster_table VALUES (2, ST_ImportFrom('rbt','/home/postgres/LC08_L1TP_113029_20191030_20191114_01_T1.TIF'));
INSERT INTO raster_table VALUES (3, ST_ImportFrom('rbt','/home/postgres/LC08_L1TP_114028_20191005_20191018_01_T1.TIF'));
INSERT INTO raster_table VALUES (4, ST_ImportFrom('rbt','/home/postgres/LC08_L1TP_114029_20200905_20200917_01_T1.TIF'));
Calculate the storage space used by the images. In GanosBase, the metadata and block data of the Raster data type are stored separately but managed in a centralized manner. The base table stores the metadata, and the block table stores the block data. To accurately calculate the data volume in the block table, you need to create a stored procedure by executing the following statements:
CREATE OR REPLACE FUNCTION raster_data_internal_total_size( rast_table_name text, rast_column_name text)
RETURNS int8 AS $$
DECLARE
sql text;
sql2 text;
rec record;
size int8;
totalsize int8;
tbloid Oid;
BEGIN
size := 0;
totalsize := 0;
sql = format('select distinct(st_datalocation(%s)) as tblname from %s where st_rastermode(%s) = ''INTERNAL'';', rast_column_name, rast_table_name, rast_column_name);
for rec in
execute sql
loop
sql2 = format('select a.oid from pg_class a, pg_tablespace b where a.reltablespace = b.oid and b.spcname=''oss'' and a.relname=''%s'';', rec.tblname);
execute sql2 into tbloid;
if (tbloid > 0) then
size := 0;
else
sql2 = format('select pg_total_relation_size(''%s'');',rec.tblname);
execute sql2 into size;
end if;
totalsize := (totalsize + size);
end loop;
return totalsize;
END;
$$ LANGUAGE plpgsql;
After creating the stored procedure, you can execute the calculation. The result indicates that the image data occupies approximately 1.2 GB of database storage.
SELECT pg_size_pretty(raster_data_internal_total_size('raster_table','rast'));
Sample result:
pg_size_pretty
1319 MB
(1 row)
Calculate the normalized difference vegetation index (NDVI). The data is stored on disks. During the initial calculation of NDVI, a mosaic operation is performed to merge the images into a complete image for NDVI analysis. The time used for the calculation is also recorded. For more information, ST_MosaicFrom.
CREATE TABLE rast_mapalgebra_result(id integer, rast raster);
INSERT INTO rast_mapalgebra_result SELECT 1, ST_MapAlgebra(ARRAY(SELECT st_mosaicfrom(ARRAY(SELECT rast FROM raster_table ORDER BY id), 'rbt_mosaic','','{"srid":4326,"cell_size":[0.005,0.005]')), '[{"expr":"([0,3] - [0,2])/([0,3] + [0,2])","nodata": true, "nodataValue":0}]', '{"chunktable":"rbt_algebra","celltype":"32bf"}');
Sample result:
INSERT 0 1
Time: 39874.189 ms (00:39.874)
Archive the block data to cold storage. Archive the block data of the remote sensing images to OSS, while keeping the metadata on disks. You need to create a stored procedure by executing the following statements to facilitate this process:
CREATE OR REPLACE FUNCTION raster_data_alter_to_oss( rast_table_name text, rast_column_name text)
RETURNS VOID AS $$
DECLARE
sql text;
sql2 text;
rec record;
BEGIN
sql = format('select distinct(st_datalocation(%s)) as tblname from %s where st_rastermode(%s) = ''INTERNAL'';', rast_column_name, rast_table_name, rast_column_name);
for rec in
execute sql
loop
sql2 = format('alter table %s set tablespace oss;',rec.tblname);
execute sql2;
end loop;
END;
$$ LANGUAGE plpgsql;
After the stored procedure is created, archive the block data to cold storage and recalculate the storage space occupied by the block data table on disks:
SELECT raster_data_alter_to_oss('raster_table', 'rast');
SELECT pg_size_pretty(raster_data_internal_total_size('raster_table','rast'));
The result shows that the storage space occupied by the block data table on disks is now 0, indicating that the block data is stored in OSS.
pg_size_pretty
0 bytes
(1 row)
Recalculate the NDVI. After archiving the block data to OSS, calculate the NDVI again:
INSERT INTO rast_mapalgebra_result select 2, ST_MapAlgebra(ARRAY(select st_mosaicfrom(ARRAY(SELECT rast FROM raster_table ORDER BY id), 'rbt_mosaic','','{"srid":4326,"cell_size":[0.005,0.005]')), '[{"expr":"([0,3] - [0,2])/([0,3] + [0,2])","nodata": true, "nodataValue":0}]', '{"chunktable":"rbt_algebra","celltype":"32bf"}');
Sample result:
INSERT 0 1
Time: 69414.201 ms (01:09.414)
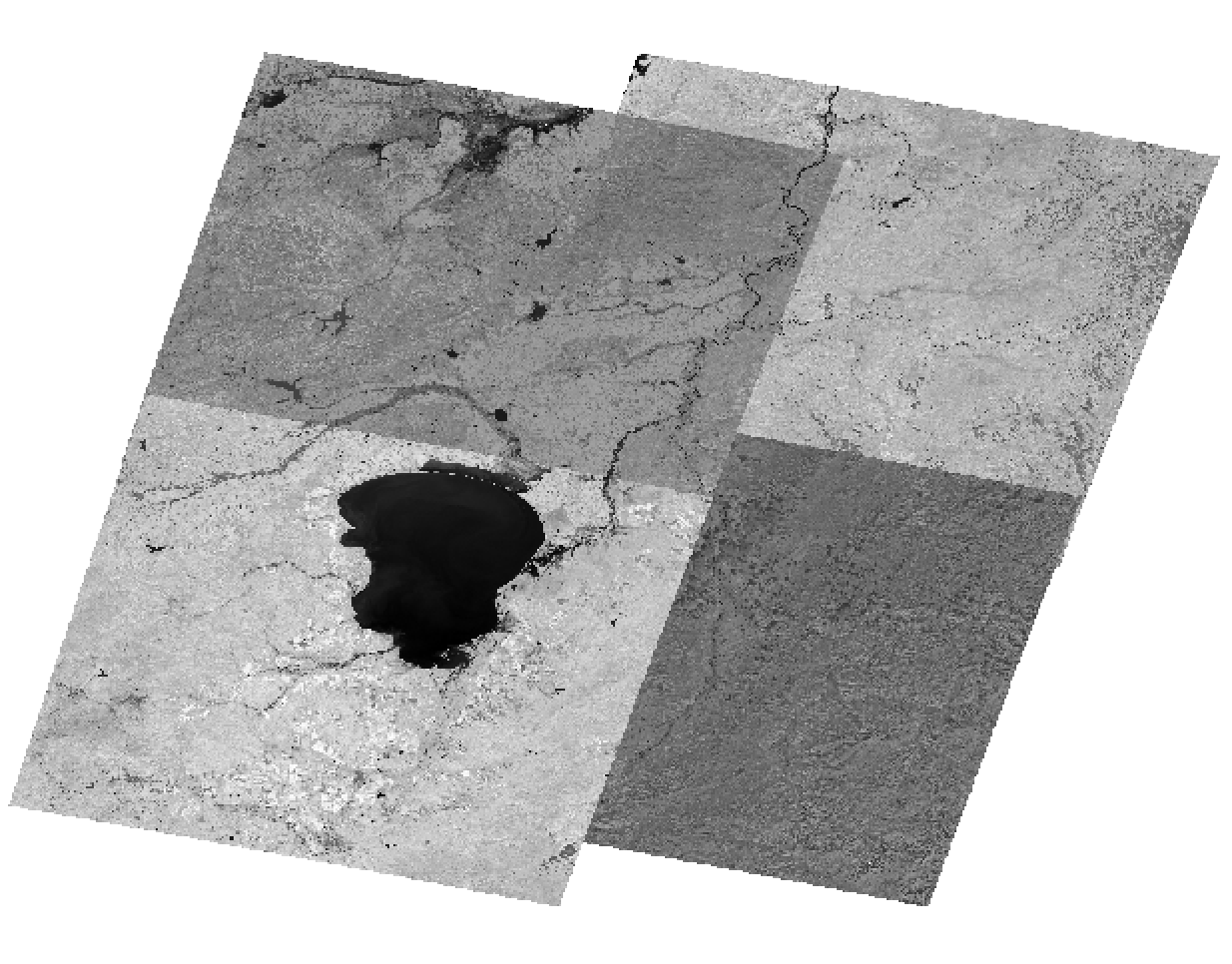
Compare storage costs and performance.
Item | Disk | OSS | Ratio |
Storage cost | 1319 MB, at USD 0.238 per GB-month, a total of USD 0.31 | 1011.834 MB, at USD 0.0232 per GB-month, a total of USD 0.023 | 10:1 |
NDVI calculation time | 39 seconds | 69 seconds | 1:1.76 |
The comparison results show that the cost of using OSS as cold storage is less than one-tenth of that of the disks. The computing performance loss is less than 100%. This is cost-effective in non-real-time statistical analysis scenarios.
Conclusion
GanosBase is used in thousands of application scenarios across numerous industries. Stability, cost-effectiveness, high performance, and ease of use are the major advantages of GanosBase. The polymorphic tiered storage feature is a core competitive advantage of GanosBase based on PolarDB for PostgreSQL (Compatible with Oracle). It offers a cost-effective, high-performance, and easy-to-use data management solution. You are welcome to try it now.