Enterprises obtain insights from vast amounts of data based on big data platforms for more timely and effective decision-making. The demand for the freshness of processed data and real-time processing capabilities are also increasing. Big data platforms commonly adopt a combination of offline, real-time, and streaming engines to meet the requirements of users for real-time performance and cost-effectiveness. However, many business scenarios require near-real-time processing at minute or hourly intervals, rather than second-level update visibility or row-level updates. Building upon its existing offline batch processing engine, MaxCompute upgrades its architecture to a near-real-time data warehouse solution. This solution integrates incremental and full data storage and management based on Delta table, enriches incremental computing capabilities, and enhances MaxCompute Query Acceleration (MCQA2.0) for second-level query responses. This topic describes the business pain points that this solution addresses and highlights the primary architectural features.
Current situation analysis
In low-timeliness business scenarios in which a large amount of data needs to be processed in batches, you can use MaxCompute to meet business requirements. In high-timeliness business scenarios in which second-level real-time data processing or streaming processing is required, you need to use a real-time data processing system or a streaming system to meet business requirements. In comprehensive business scenarios, such as the combination of minute-level or hour-level near-real-time data processing and batch processing of large amounts of data, specific issues may occur regardless of whether you use a single engine or multiple federated engines.
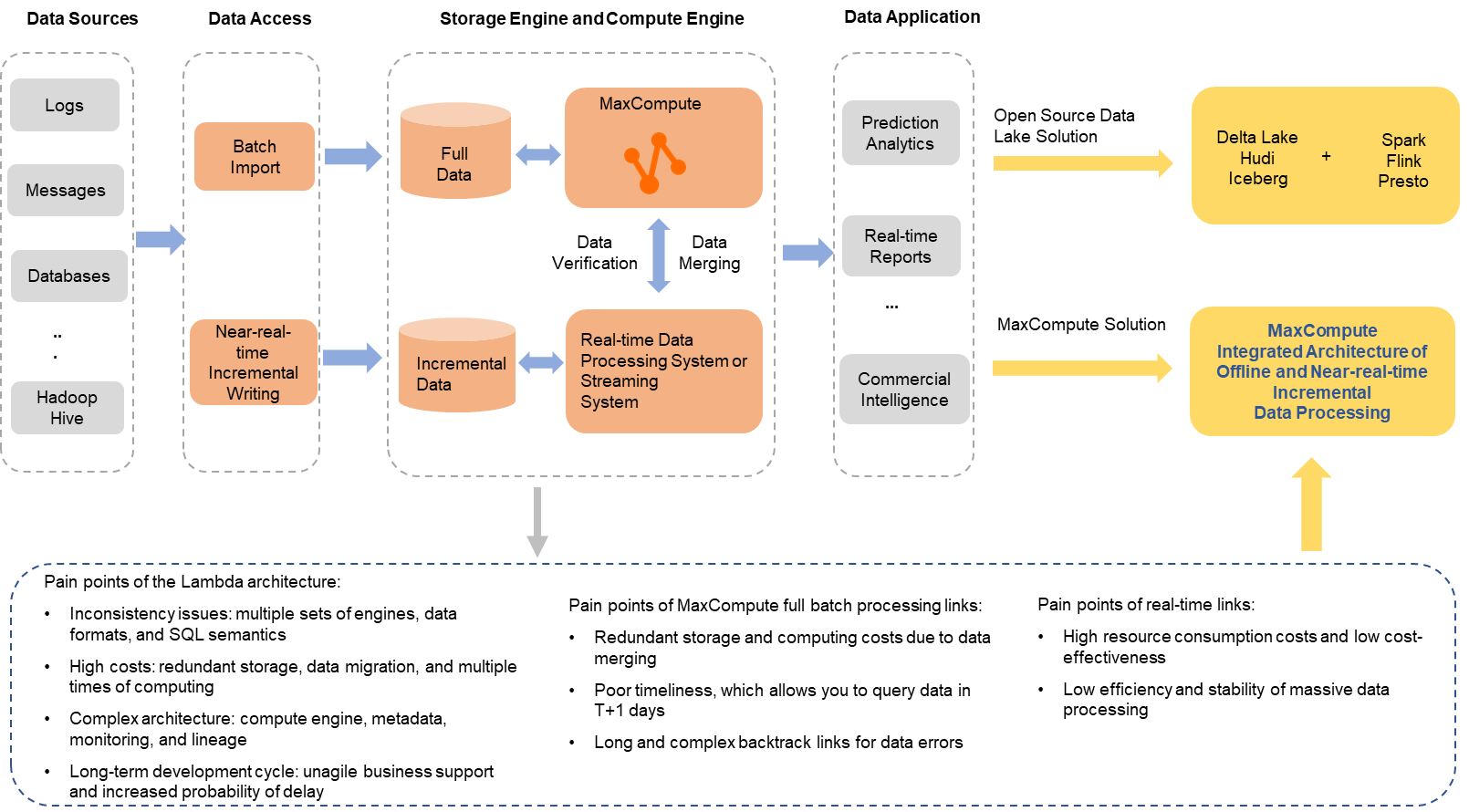
Specific issues may occur if you use only MaxCompute for batch processing in specific scenarios, as shown in the preceding figure. For example, if you use MaxCompute in scenarios in which minute-level incremental data and full data of users need to be continuously merged and stored, additional computing and storage costs are generated. If you use MaxCompute in scenarios in which complex data processing links and processing logic need to be converted into batch processing of data within T+1 days, the complexity of data processing links increases and the timeliness cannot meet business requirements. If you use only a real-time data processing system in the preceding scenarios, the resource costs are high, the cost efficiency is low, and the batch processing of large-scale data is unstable. In most cases, the Lambda architecture is used as a solution. In the Lambda architecture, MaxCompute is used for batch processing of full data, and a real-time data processing system is used for incremental data processing to meet high timeliness requirements. However, the Lambda architecture can cause known issues, such as data inconsistency between multiple sets of processing and storage engines, additional costs due to redundant storage and computing of multiple copies of data, a complex architecture, and a long-term development cycle.
To address the preceding issues, the big data open source ecosystem launched various solutions in recent years. The most popular solution is that the open source data processing engine Spark, Flink, or Presto is deeply integrated with the open source data lakes Hudi, Delta Lake, and Iceberg to implement a unified compute engine and data storage. This solution can help resolve a series of issues caused by the Lambda architecture. An incremental data storage and processing architecture is developed based on the architecture of MaxCompute. The architecture provides an integrated solution for batch data processing and near-real-time incremental data processing. The architecture maintains the cost-effectiveness of batch processing and meets the business requirements for minute-level incremental data reading, writing, and processing. The architecture can also provide practical features, such as the UPSERT operation and the time travel feature, to expand business scenarios. This helps reduce data computing, storage, and migration costs and improve user experience.
MaxCompute near-real-time architecture
In the new architecture, MaxCompute supports various data sources to allow you to easily import incremental and full data to a unified storage system by using customized access tools. The backend data management service automatically optimizes the data storage structure. A unified computing engine is used to support near-real-time incremental data processing and batch processing of large-scale data. A unified metadata service is used to support transaction management and file metadata management. The new architecture provides multiple benefits, including resolving issues that occur when only a batch processing system is used, such as redundant computing and storage and low timeliness, preventing the high resource consumption of real-time data processing systems or streaming systems, eliminating data inconsistency between multiple sets of systems in the Lambda architecture, and reducing the redundant storage cost of multiple copies of data and the cost of data migration between systems. The SQL Optimizer is optimized for incremental queries, particularly in materialized view refresh scenarios. The optimizer selects either a state-based incremental algorithm or a table snapshot-based incremental algorithm based on cost estimation for the refresh operation. The MaxCompute query acceleration 2.0 (MCQA2.0) enhances query performance and stability based on a strongly isolated resource foundation of virtual workloads (VW). Relying on the self-developed fast data cache (FDC), the acceleration layer optimizes the entire cache chain. The optimizer adds a latency optimization mode, and the runtime optimizes vectorized execution to avoid overhead during execution. The end-to-end integrated architecture can meet the business requirements for computing and storage optimization of incremental data processing and minute-level timeliness, ensure the overall efficiency of batch processing, and effectively reduce resource costs.
Core features
The MaxCompute near-real-time data warehouse offers three main features: MC Delta Table for minute-level data import, incremental computing capabilities that balance latency and throughput, and the enhanced MCQA2.0 for second-level query responses.
The core features include:
Delta Table incremental table format: uses the AliORC file format, supports minute-level data imports and UPSERT operations. It offers standard Change Data Capture (CDC) methods for reading and writing incremental data. It relies on storage service of MaxCompute and metadata service for automatic data management.
Incremental computing: based on the Delta Table format, MaxCompute introduces incremental computing features such as incremental materialized views, Time Travel, and Stream Table. Incremental materialized views and scheduled tasks offer different trigger frequencies, providing users with options to balance latency and throughput.
MCQA 2.0 query acceleration: an upgrade to MaxCompute Query Acceleration, it enhances performance stability through a strong isolation environment and extends support from DQL SELECT queries to full SQL functionality, including DDL and DML. Performance is further improved through full-chain caching and asynchronous optimization.
These features are built on the original SQL engine of MaxCompute, which allows users to analyze vast amounts of data more cost-effectively without altering their development practices.
Benefits
To support the business scenarios and business migration of the open source data lakes Hudi and Iceberg, the new architecture provides specific common features. The self-developed new architecture also provides the following benefits in terms of features, performance, stability, and integration:
Provides a unified design for storage, metadata, and compute engines to achieve in-depth and efficient integration of the engines. The new architecture provides the following benefits: low storage costs, efficient data file management, and high query efficiency. In addition, a large number of optimization rules for MaxCompute batch queries can be reused by time travel and incremental queries.
Provides a full set of unified SQL syntax to support all features of the new architecture. This facilitates user operations.
Provides in-depth customized and optimized data import tools to support various complex business scenarios.
Seamlessly integrates with existing business scenarios of MaxCompute to reduce migration, storage, and computing costs.
Supports automatic management of data files to ensure better read and write stability and supports automatic optimization of storage efficiency and costs.
Is fully managed on MaxCompute. You can use the new architecture out-of-the-box without additional access costs. You need to only create a Delta table to use the features of the new architecture.
Is a self-developed architecture. You can manage data development for your business requirements based on the new architecture.